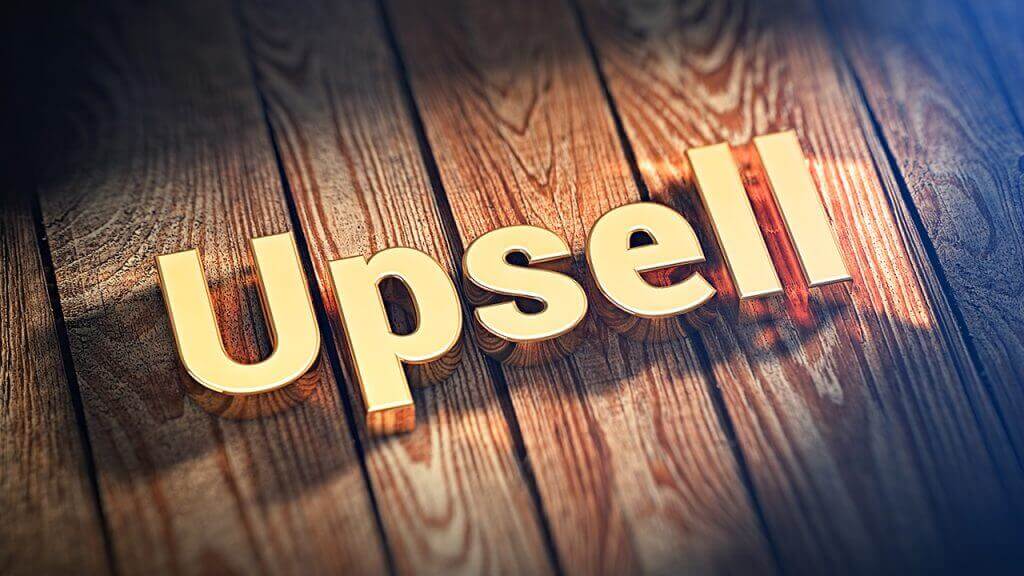
Upselling has traditionally been a guessing game. Trial and error. In fact, an article from not all that long ago suggested that the front desk should “gauge the needs of callers and walk-ins” then offer relevant upselling opportunities.
NB: This is an article from Nor1
In effect, asking the front desk to do something they aren’t really meant to do nor have the time to do, not on their own, at least. Laurie R. Santos and Alexandra G. Rosati of Yale University noted that humans “consistently attend too much to irrelevant information, fall prey to contextual and situational variables, and even rationalize our bad decisions. Moreover, many of these irrational biases operate quickly, effortlessly, and outside of our awareness” (NCBI). It’s not that we’re poor decision-makers; we’re just occasionally inaccurate and sometimes slow to come to the right conclusion.
What the front desk needs is not better decision-making skills or even training on how to upsell better. Instead, the front desk requires a tool that fortifies their decision making (offer and price), so they can focus on engaging the guest. Machine learning, or ML, does this. ML technology is critical to upselling because it takes the guesswork out of what to offer, when to offer it, and at rate that will optimize revenue. At arrival, the still essential front desk agent focuses instead on creating the ideal guest service experience.
ML brings both precision and dynamism to the upselling process, as opposed to static offers with flat rates. Upselling with ML uses millions of data points to generate offers that each guest is most likely to find attractive and, thus, accept. These decisions are generated real-time, across the reservation life-cycle —from booking through post-stay, across digital and in-person touchpoints.
With ML-generated offers, guests can receive multiple and different opportunities to enhance their stays based on their reasons for travel and personal preferences. The benefits go further. For hotels, upselling as an estimation of what the guest wants has not been a reliable form of revenue, but more like an undependable bonus. Rather than being a bonus (e.g., a nice-to-have), machine-learning generated upselling can provide predictable, forecastable revenue, with upgrade pricing varying based on the guest, bringing in the maximum possible revenue while optimally balancing the house. No more flat-rate offers that don’t capitalize on perishable inventory, or worse, giving complimentary upgrades because standard rooms are oversold.
The precision and dynamism offered by ML cross over three key areas: selection, presentation, and pricing.
1). Offer Selection
Hotels have so much more to offer than an upgrade to the next room category. Room location, views, balconies—all of these things are attributes of value to guests. Further, we know beyond a shadow of a doubt that guests will pay for early check-in and late checkout, along with other non-room inventory products and services.
Here’s where ML comes in. Not every guest needs to see every offer available at the property. This approach is counter-productive and creates decision fatigue and rather than increasing the chances the guest will choose an upgrade, they walk away. Machine learning chooses what to offer the individual guest based on hundreds of data points, including the following:
- What’s most relevant to the guest based on reason for travel or guest preferences
- What products and services are available at the hotel at a given point in the reservation life-cycle.
- How offers have performed in the past
- How guests similar to this one have interacted with offers like this one
- How this particular guest has engaged with offers at this property earlier in the reservation life-cycle.
We call this “true personalization,” that is, in real time, combining relevant guest data with merchandising performance data to ensure the right offer is made to the right guest at the right price. With ML generated offers, hotels move from static suggestions, like the suite upgrade, to a dynamic set of opportunities tailored to the guest. It relies not just on what has been booked, but also on what this guest—or guests just like her—have NOT booked. Negative response information is equally as crucial as positive information for ML algorithms. Having both negative and positive information makes the resulting recommendations far more accurate, which increases the probability the guest will opt for one or more of the featured upgrades selected just for her.
2). Priority Presentation
ML goes a step further than determining which offers are presented to which guest. The algorithms determine in what order the offers should be presented and delivered. The old static method of presentation shows upgrades in no particular order (or in an arbitrary order chosen by the property). With ML, offer assortment (which products appear together and in what order) is a significant driver of conversion, leveraging the buyer behavior dynamics of anchor and reference pricing.
Further, the optimal number of offers will be presented at any time to avoid the paradox of choice. That said, the offers for each guest will change based on where the guest is in the purchase pathway. As an example if a guest has received an offer at booking and has chosen not to book it, the offer likely won’t be presented again at check-in, but a new offer will replace it.
3). Dynamic Pricing
Historically, a hotel decided to offer a suite upgrade for, let’s say, a static $70 or whatever the revenue manager determined made sense on a given day or week or month. The front desk, given instructions on the rate, gave each guest who walked through the door the same upgrade opportunity with the same static price. Maybe the offer would be distributed via email, too, again with the same pricing and no real consideration about guest preferences.
ML changes the game in a big way by using dynamic pricing, determining at what rate the offer should be presented. ML generated upselling correlates and weights many data points to determine at what rate an offer should be priced, including:
- Booked rate
- Room type delta
- Current rates
- Guest demand
- Room availability
Based on these considerations, and many others, the algorithm decides precisely at what rate the guest is most likely to book the upgrade while still allowing the property to achieve the greatest revenue.
What this precision and dynamism equate to is true personalization, meaning an offer is made real-time, specific to a guest, and based on that guest’s interests, geography, point in the reservation life-cycle and particular purpose of travel. Personalization is a progression that evolves from making context-based decisions to a micro-segmented perspective of the guest and, ultimately, to the specific guest based on that individual’s preferences, clicks, and real-time interaction through the reservation life-cycle.
As the system receives feedback from a guest during the reservation life-cycle, it has a greater number of data points with which to correlate and decision on. Personalization at this level is correlating many different things about a guest all at the same time so that it knows what combination of attributes that guest values, at what price, and at what point the guest will be most likely to upgrade or add on service or attributes. An ML platform surfaces this intelligence during every relevant interaction with the guest.
Conclusion
A recent trend has been to create new features to upsell guests. Deliver exercise equipment to guest rooms on demand. Install dream mattresses in some rooms for an extra fee. Some hotels are charging for higher thread-count sheets, an in-room mini-bar, and nicer shampoo. An alternative to creating upselling opportunities is to do a better job at selling what’s already available. The first step is to implement exacting and dynamic tools that will make the most of what the hotel has available—and still provide all guests with the same basic levels of comfort and amenities. Before creating new tiers of experience, hotels should understand what guests are already willing to pay for and at what price. Only machine learning technology can truly accomplish this.